I’m always fascinated by what one – just one – motivated person can do. Sure, results usually require teams, but there is always that one standout personality that drives that result. As you’re about to see and read, Kavya Kopparapu is one of those remarkable people.
At just 16 years of age, Kavya has developed a readily accessible, very inexpensive way to diagnose diabetic retinopathy. Diabetic retinopathy is the most common cause of vision loss among people with diabetes. It’s a leading cause of blindness among working-age adults accounting for around 8% of all blindness in the USA alone. Yet early diagnosis can result in total cure!
552 million of us will be diabetic by 2030!
According to The International Diabetes Federation, more than 400 million adults currently have diabetes. Consider these statistics (2015):
- One out of every 11 adults has diabetes
- One in two of those (46.5 per cent) is undiagnosed
- About 35% will develop diabetic retinopathy, leading many to preventable blindness
- In 2015, 12 per cent of global health expenditure went on diabetes (USD$673 billion)
- 542,000 children have type 1 diabetes
- Every six seconds a person dies from diabetes
What is really interesting (to me, at least), is that Kavya is not your typical ‘genius’ or computer ‘nerd’. She is simply one person who saw a need and decided to do something about it…
When 16-year-old Kavya Kopparapu wasn’t attending conferences, giving speeches, presiding over her school’s bioinformatics society, organizing a research symposium, playing piano, and running a non-profit, she worried about what to do with all her free time.
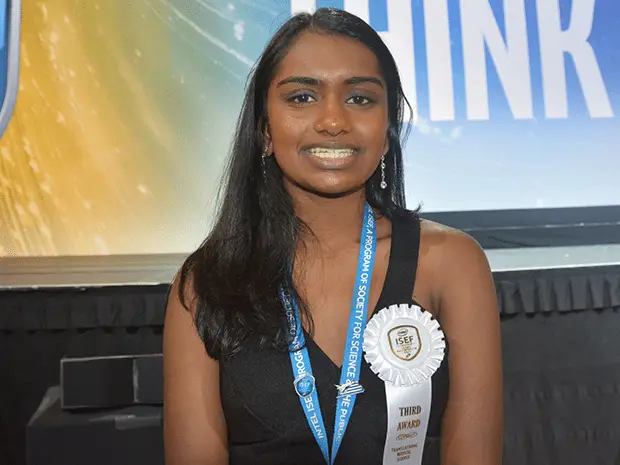
It was June 2016, the summer after her junior year in high school, and Kopparapu was looking for a new project that would use her computer science skills. Her thoughts quickly turned to her grandfather, who lives in a small city on India’s eastern coast.
In 2013 he began showing symptoms of diabetic retinopathy, a complication of diabetes that damages blood vessels in the retina and can lead to blindness. Eventually he was diagnosed and treated, but not before his vision deteriorated. Still, he was lucky: Although treatments such as medication and surgery can stop or even reverse eye damage if the disease is caught early, most patients never receive care.
Kopparapu knows the statistics by heart: Of 415 million diabetics worldwide, one-third will develop retinopathy. Fifty percent will be undiagnosed. Of patients with severe forms, half will go blind in five years. Most will be poor.
“The lack of diagnosis is the biggest challenge,” Kopparapu says. “In India, there are programs that send doctors into villages and slums, but there are a lot of patients and only so many ophthalmologists.” What if there were a cheap, easy way for local clinicians to find new cases and refer them to a hospital?
That was the genesis of Eyeagnosis, a smartphone app plus 3D-printed lens that seeks to change the diagnostic procedure from a 2-hour exam requiring a multi-thousand-dollar retinal imager to a quick photo snap with a phone.
Kopparapu and her team—including her 15-year-old brother, Neeyanth, and her high school classmate Justin Zhang—trained an artificial intelligence system to recognize signs of diabetic retinopathy in photos of eyes and offer a preliminary diagnosis. She presented the system at the O’Reilly Artificial Intelligence conference, in New York City, last month.
“The device is ideal for making screening much more efficient and available to a broader population,” says J. Fielding Hejtmancik, an expert in visual diseases at the National Institutes of Health (NIH). Other research groups, including Google and Peek Vision, have recently announced similar systems, but Hejtmancik is impressed with the students’ ingenuity. “These kids have put things together in a very nice way that’s a bit cheaper and simpler than most [systems designed by researchers]—who, by the way, all have advanced degrees!”
Kopparapu has always had a scientific mind. Growing up in Herndon, Virginia, she and her brother built K’nex creations, watched MythBusters and Cosmos, and read Scientific American together over breakfast. But she didn’t get hooked on computers until she attended a programming workshop run by the National Center for Women and Information Technology.
“I went home and taught myself Java, HTML, Python, C,” she says. “My mom had to tear me away from the computer. I’d forget to eat.”
In high school, she took classes on computer science, then computer vision, then artificial intelligence—but she was troubled to realized that in each class, she was one of only a few girls. She resolved to start an organization to empower girls to pursue computer science.
“I don’t think the problem is a lack of passion,” she says. “It’s more ‘I don’t feel like I’m good enough.’”
She founded the Girls Computing League, wooed sponsors such as Amazon Web Services and the president of Harvey Mudd College, and now puts on coding workshops for underprivileged kids.
Eyeagnosis began as most endeavors do these days. “I googled a lot,” Kopparapu says. She also sent a lot of emails—to ophthalmologists, computational pathologists, biochemists, epidemiologists, neuroscientists, physicists, and experts in machine learning. Then she put together a plan.
First her team worked on the diagnostic AI, choosing to use a machine-learning architecture known as a convolutional neural network (CNN). Neural nets are behind the recent explosion in artificial intelligence, including advances in speech recognition, machine translation, and image captioning. They acquire these skills by parsing vast sets of data (millions of photos of cats, say) and looking for patterns of similarity.
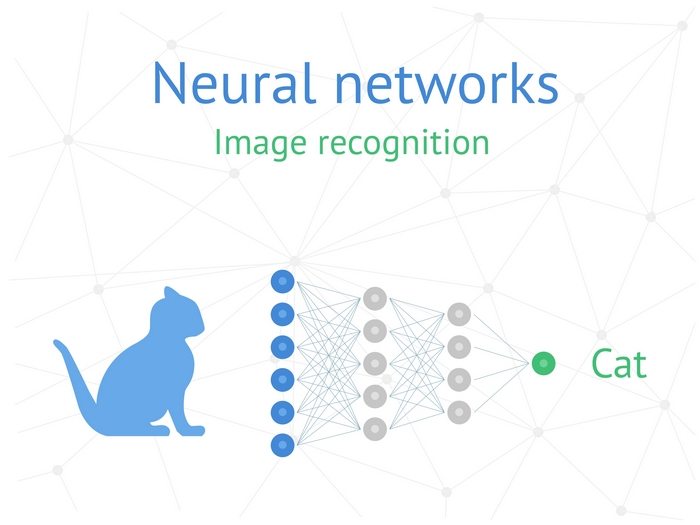
CNNs are especially good at classifying images, so it’s no coincidence that their design resembles the brain’s visual system. Information passes through hierarchical layers of “neurons” called nodes; with each layer, the network recognizes ever more abstract features: Pixels become edges become shapes become objects.
“It’s kind of funny that we’re using a system based on how the retinal system works to diagnose a retinal disease,” Kopparapu says.
Rather than build a network from scratch, she chose an off-the-shelf model developed by Microsoft researchers called ResNet-50. But in order to teach the system to recognize an eye disease, she needed training data.
She found that data in the NIH’s EyeGene database, which included 34,000 retinal scans. Many of these images, taken under various conditions with different types of cameras, were blurry or poorly exposed. “But that was actually a good thing,” Kopporapu says. “It’s very representative of the real-world conditions you’d get with using a smartphone.”
By August 2016, her team had trained ResNet-50 to spot diabetic retinopathy with the accuracy of a human pathologist. By October, she had made arrangements with Aditya Jyot Eye Hospital, in Mumbai, to test the Eyeagnosis app, which not only detects disease but also highlights blood vessels and microaneurysms in an image—a process that normally involves injecting a fluorescent dye into patients’ blood.
“We’re trying to make it as easy as possible for an ophthalmologist to look at all that info and say ‘Here’s my final diagnosis.’”
In November, she shipped her first 3D-printed prototype for the system’s lens to the hospital. When fitted onto a smartphone, the lens focuses the phone’s diffuse, off-centered flash to best illuminate a retina. The complete Eyeagnosis system has already been tried on five patients at the hospital, and in each case it made an accurate diagnosis.
Hejtmancik, the NIH expert, notes that there’s a long road to clinical adoption. “What she’s going to need is a lot of clinical data showing that [Eyeagnosis] is reliable under a variety of situations: in eye hospitals, in the countryside, in clinics out in the boonies of India,” he says.
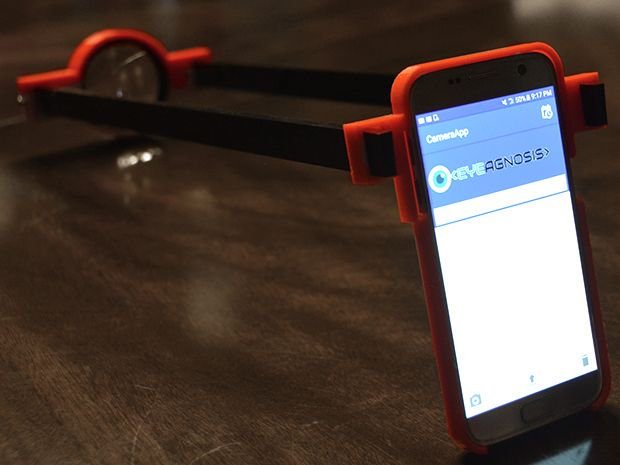
The Eyeagnosis system uses a 3D-printed lens and an AI-enabled smartphone app to diagnose diabetic retinopathy.
Still, Hejtmancik thinks the system has real commercial potential. The only problem, he says, is that it’s so cheap that big companies might not see the potential for a profit margin. But that affordability “is exactly what you want in medical care, in my opinion,” he says.
This article was originally published by Ariel Bleicher in IEEE Spectrum.
BTW, have you ever wondered why there are so many smart Indian and Chinese kids? Have you noticed how many CFO’s, CEO’s and COO’s of great ‘start-ups’ come from those two cultures? The answer is simpler than it may appear:
One in every three people on this planet is either Indian or Chinese!
Yes, perhaps their cultural backgrounds drive them to work harder and even, work smarter. But, on average, they are no more intelligent than any other race or culture. It’s just that statistically, there are a lot more people of Indian and Chinese descent. To put that in perspective, there are about 150 million more Indians under 18 than the total American population!
Feeling inspired? Kavya may seem exceptional, but you and I can also make a real difference!